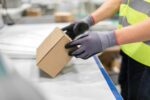
Visit Our Sponsors |
|
|
|
|
|
|
|
|
|
|
|
|
|
|
|
|
|
|
|
|
|
|
|
|
|
|
|
|
|
|
|
|
|
|
|
|
|
|
|
|
|
|
|
|
|
|
|
|
|
More and more organizations around the world are turning their eyes away from decision making processes, based on single-point estimates and viewing their risks and opportunities with more sophisticated techniques. One such technique is Monte Carlo simulation (MCS). In a nutshell, MCS allows the examination of all the possible outcomes of decisions and assesses the impact of risk, allowing for better decision making under uncertainty. It is a computerized mathematical technique that allows the business modeler to account for risk in quantitative analysis and decision making. MCS furnishes the decision-maker with a range of possible outcomes and the probabilities they will occur for any choice of action.
How Monte Carlo Simulation Works
MCS performs risk analysis by systematically building models of many possible results. It does this by substituting a range of values - a probability distribution - for any factor that has inherent uncertainty.
Consider a case of a company trying to forecast inventory demand based on sales of a new product in a new market in order to quantify its potential profitability. A traditional approach would only consider single-point estimates, or in a better case, an analysis of three scenarios - the "conservative", "most likely" and "optimistic" cases. With MCS, however, the modeler can choose a single probability distribution function embedded in an Excel-based model to represent all possible points for inventory demand.
A pert probability distribution function, for example, allows the modeler to use an expert's best opinion of the traditional three point estimates: the minimum, maximum, and most likely values. These three parameters are used to build a continuous representation of all possible actual scenarios. Assume that such a demand could be built defining a minimum value of 100 units, a maximum value of 250 units and a most likely value of 130 units. A graphical representation of such a pert probability distribution would render a curved shape. Using the resulting pert distribution, probabilities can now be easily calculated and validated by an expert. The expert could then assess such a probabilistic view by validating that, for example, a three-percent probability of exceeding a demand larger than 200 units exists and an 18.5 percent probability of 120 units or less exists.
MCS would then calculate the resulting profitability over and over, each time using a different set of random values from the input probability functions, like the pert distribution for inventory levels. Depending upon the number of uncertainties and the ranges specified for them, an MCS could involve thousands or tens of thousands of recalculations before it is complete.
Consider a case in which the modeler has inserted uncertainty into a relatively large quantity of variables. Variables such as sales demands, material costs, investment outlays, delivery and processing times, inventory uncertainty and, of course, disaster risk can be modeled into a spreadsheet that considers both independent and interrelated variables. By using probability distributions, variables can have different probabilities of different outcomes occurring, thus greatly enhancing model accuracy.
Consider another example, this time modeling disaster risk, a typical event risk. First of all, an assessment of the probable frequency of such an event must be made. Then, the magnitude (usually expressed financially) of such an event must be incorporated.
Assume that the modeler wants to incorporate into the analysis the total impact of a supplier's major inventory interruption. Experts have advised what they might define to be "major" as an event in which a supplier fails to supply inventories for one week or more. They have also assessed that such an unlikely event might probably occur once every five years. The magnitude of such a disruption would escalate from $1m up to $5m, with a most likely outcome of $2m.
To account for the dual frequency/magnitude factors, a "compounded" distribution function could be built that incorporates uncertainty both for the frequency of occurrence and the impact the stoppage has when it does occur. When MCS is run, the model will consider thousands of scenarios. Once every five years, on average, a scenario will appear in which such a disruption will occur, considering a magnitude of event varying from $1m to $5m, with a most likely outcome of $2m.
Depending on the model's intention, being a tactical or strategic decision, it needs to be able to answer questions like: "What is the probability this critical part will arrive on time?" and "What are the chances of failure at this point in the process?", or "What is the profitability of entering into a new regional market, provided likelihoods of major vendor disruptions and major uncertainties of demand?"
Other variables with possible variation such as prices, material costs and overhead expenses can be considered. For each of them, the modeler can obtain expert opinion or, preferably, use historical data to find distribution functions that best describe that data. Software is available that can "fit" many different types of statistical distributions to data sets. By using a fitting technique, the modeler is able to represent the reality of historical data and incorporate its "shape" and "dimension" back into the model.
Consider an MCS executing 10,000 iterations or scenarios. The model is recalculated 10,000 times considering all uncertainties previously inserted: prices, costs, unit sales and of course, likelihoods of major supplier disruption. For each one of these scenarios, MCS picks different samples from each uncertain input distribution.
After the simulation, assume an "average" profit outcome is positive with a mean of about $700. But, there exists a relatively high chance - over 30 percent - that a negative outcome where money is lost may occur. On the other hand, a "high" positive outcome is also possible: there exists more than a 20-percent likelihood of obtaining $2,000 or more. Such a curve also reveals the relatively wide amplitude of varying possible outcomes. The standard deviation of such a profit - $1,777 - is more than 2.5 times the mean expected outcome. This tells us there is significant risk in the venture.
Knowing this a decision maker wants to determine which particular variable has the largest impact upon the expected profit out of all the possible variations of this venture?
A tornado chart may be able to answer such a question. Such a chart provides a measure of how much the output would vary as each of the various inputs change during simulation. It is a representation of how much variation in profit is accounted for by each input variable. In this example, supplier failure could be the variable which has the largest impact upon profit.
This approach allows decision-makers to perform trade-off analysis among expected costs, quality acceptance levels and on-time delivery distributions. It also provides alternative tools to evaluate and improve supplier selection decisions in an uncertain supply chain environment.
Risk analysis utilizing Monte Carlo simulation assists organizations that rely heavily on supply chain efficiency to forecast any number of potential obstacles that could cause a disruption in continuity. Additionally, knowledge of the probability that those risks may occur further empowers decision-makers to formulate contingency plans, meet customer demand on time, and ultimately increase bottom line profitability.
Source: Palisade Corporation
Keywords: supply chain risk management, supply chain management IT, value chain IT, supply chain solutions, business disruption contingency planning
RELATED CONTENT
RELATED VIDEOS
Timely, incisive articles delivered directly to your inbox.